Why Smarter Data is Better Data
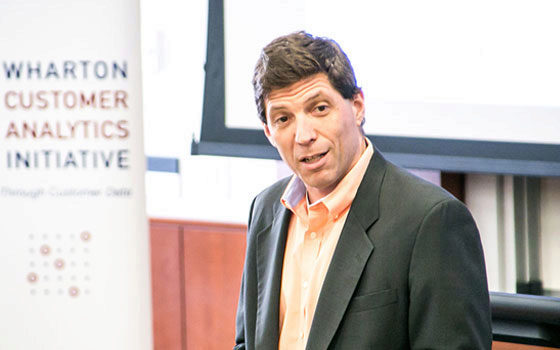
AI and Analytics for Business, together with Teradata, co-sponsored an event that gathered an intimate crowd of high-level industry practitioners to discuss why smarter, smaller datasets should be one of the highest priorities behind a company’s customer analytics best practices. AIAB Co-Directors, Eric Bradlow and Pete Fader, with Teradata’s Chief Analytics Officer, Bill Franks presented and moderated a discussion surrounding intelligent data compression and how nimble datasets can help lead companies to a better understanding of their customers and their organization.
Why is smaller smarter?
More and more companies are jumping on the big data bandwagon — collecting petabytes of data to gain a comprehensive understanding of their customers. Yet, as Professor Eric Bradlow joked, “No one throws a party when someone announces they have petabytes of data.”
Large datasets inherently come with outrageous computation times. It’s inefficient to run models because there are so many numbers to crunch. Data scientists often spend days running models on enormous datasets and the results aren’t what was expected and now the model needs to be adjusted, or the wrong columns were pulled, etc. It’s a long and inefficient cycle.
To break this cycle, this is where statistical sufficiency comes in: use the smallest amount of information you need to yield accurate results and “get rid of the rest”.
How can datasets be compressed?
To compress your data, two (of the many options) are: (1) to break it up by deconstructing the data into smaller datasets and then recombining at the end, and (2) knowing when old data is too old to be useful and to “prune it off”.
For example, make a 10,000,000-row table into 10,000 “mini datasets” then run the model on each of them. The result is that the model has been fit a thousand times, in much less time with the added bonus of an uncertainty estimate. There are now 1,000 results from running the same model once over one thousand smaller data sets.
It’s also important to know when to get rid of data that isn’t relevant anymore. For example, if a dataset contains records from 1960, it’s (likely) not important that data be included in the models because modern customer behavior has nothing to do with customer behavior or purchasing behavior in the 1960’s.
To determine the point at which the data is no longer useful, fit the data incrementally. Run the model for the last three years, then five, then eight years and keep testing until the answer changes. When the answer changes, that’s the point when the older data may be left out, leaving only the relevant customer data.
Why “C” is Eric Bradlow’s favorite letter of the alphabet
With extraneous data removed from the table, data is easily handled. For marketers, it also makes patterns, or stories in the data easy to find.
Marketers like to compress customer data, when valuing customers, down to three numbers: R,F, and M (recency, frequency, and monetary value, i.e. the amount the customer spent). When combined, they tell marketers which customers are the best ones by determining customer buying patterns over time. The catch is that RFM assumes customers are consuming at a constant rate. But marketers know that isn’t true for all goods. For example, people who shop at Amazon, or binge on video content aren’t consuming at a constant rate. And thus, RFM isn’t enough on its own to determine accurate purchase behavior. It’s missing an important facet, C = “clumpiness.”
The results of this research is two-fold. It proves that customers who binge-buy, aren’t dead (or inactive as customers) between binging periods, and it proves that clumpy customers spend more over future time periods, all else equal. You can read about clumpiness in depth, here.
If the horizontal lines represent time and the squares represent transactions, the Customer B is considered clumpy. In other words, they are not buying at a constant rate which means that RFM alone can’t provide the most accurate summary information about this customer. There’s a fourth number (clumpiness is actually a score between 0 and 1) that should be computed: “C.” This number accounts for consumers who are not consuming at a constant rate which can give marketers a better understanding of when the next “binging period” might begin.
Clumpiness is an excellent tool for two reasons: It’s easy to compute (read how to calculate clumpiness here) and it can provide an insight to Customer Lifetime Value (CLV). For example, if an organization calculates each customer’s clumpiness score they can rank them from most to least clumpy and since the clumpiest customers are the best customers, organizations could have an account of which customers have the highest lifetime value.
Smart datasets and Corporate-Based Company Valuation
Broadly, Customer Lifetime Value (CLV) is a prediction of the net profit attributed to the future relationship with a customer. Which, according to Pete Fader is the starting point for determining any organization’s total value.
Companies typically determine net worth from the top down (or, the “traditional way”). As Professor Fader put it, “We look at a bunch of financial stuff to figure out the total value of a company.” However, organizations should consider valuing the organization from the bottom up. Taking available data from companies (the aforementioned “financial stuff”) and augmenting it with customer data. You can read about how to actually compute this, here.
It’s one of the newer and more exciting applications of CLV. It’s the idea behind calculating the value of the entire corporation from the bottom up. Simply put, every dollar that an organization earns is through its customers. If we set aside some of the non-operational assets, firms can add up all the individual customer lifetime values — and the ones likely acquired in the future — to determine the total value of the organization. Bill Franks said, “After today, I think my new catch phrase is, ‘customers are assets in the literal sense of the word.’”
In Sum
Big Data is powerful; it’s rich with invaluable customer data that can be leveraged into actionable insights that can drive profits and change how businesses make decisions. But to find the most accurate story beneath the data, analyses should be done on smaller, smarter data sets. It’s in these windows that the clearest snapshots of customers are found.
The event resulted in a great dialogue with the audience that left the presenters inspired to explore new research paths – and possibly a book. Following the event, they sat down to talk about their “take-aways” from the day in this video.