Artificial Intelligence Risk & Governance
By Artificial Intelligence/Machine Learning Risk & Security Working Group (AIRS)
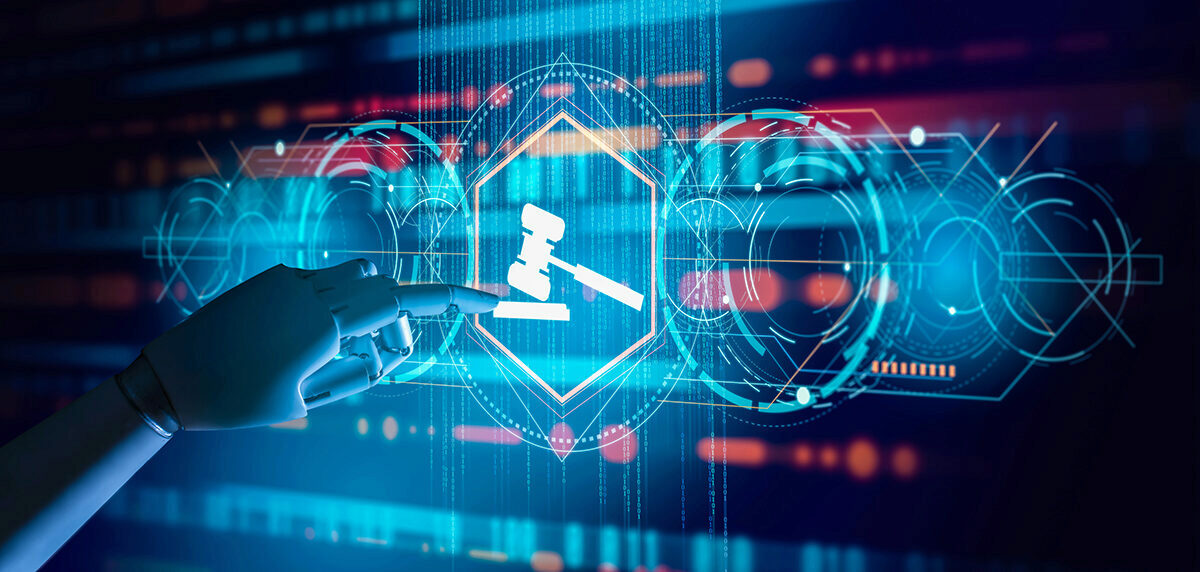
Executive Summary
As financial services firms evaluate the potential applications of artificial intelligence (AI), for example: to enhance the customer experience and garner operational efficiencies, Artificial Intelligence/Machine Learning (AI/ML) Risk and Security (“AIRS”)[1] is committed to furthering this dialogue and has drafted the following overview discussing AI implementation and the corresponding potential risks firms may wish to consider in formulating their AI strategy. This white paper provides AIRS’s views on potential approaches to AI governance for financial services including potential risks, risk categorization, interpretability, discrimination, and risk mitigation, in particular, as applied to the financial industry.
This paper is intended for discussion purposes only and is not intended to serve as a prescriptive roadmap for implementing AI/ML tools or as a comprehensive inventory of risk associated with the use of AI. Readers are encouraged to consider the information provided in this paper for reference and discussion purposes. They should assess, implement, and tailor their firms’ AI/ML programs and respective controls as appropriate for their business model, product and service mix, and applicable legal and regulatory requirements.
The views expressed in this paper are those of the individual contributors and do not constitute the views of any of the firms with which the contributors are associated or by which they are employed.
Key Takeaways
- AIRS believes there are significant potential benefits of AI and that its adoption within financial services presents opportunities to improve both business and societal outcomes when risks are managed responsibly.
- This paper explores the potential risks of AI and provides a standardized practical categorization of these risks: Data Related Risks, AI/ML Attacks, Testing and Trust, and Compliance
- AI governance frameworks could help organizations learn, govern, monitor, and mature AI adoption. Four core components of AI governance are: definitions, inventory, policy/standards, and a governance framework, including controls.
- AI, in certain use cases, could lead to privacy issues, and/or potentially discriminatory or unfair outcomes, if not implemented with appropriate care. We explore, in detail, the subject of interpretability and discrimination in using AI for certain use cases.
- While there is no one-size-fits-all approach, practices institutions might consider adopting to mitigate AI risk include oversight and monitoring, enhancing explainability and interpretability, as well as exploring the use of evolving risk-mitigating techniques like differential privacy, and watermarking, among others.
AIRS Next Steps
This document is meant to be the first of several iterations and further contributions from the AIRS group. These insights are based on collective experience of AIRS, and the suggestions we outline are, as a result, not meant to be comprehensive. AIRS plans to continue to build and engage an active community on these issues. Contact information is provided in Section 6 (Acknowledgments) if there is any feedback or if readers wish to comment on this paper or AIRS.
1. Overview
1.1 Document Purpose & Scope
The business uses, regulatory interest and research in artificial intelligence and machine learning (AI/ML) have seen an exponential increase over the last few years. Discussions regarding the use of Artificial Intelligence (AI) and Machine Learning (ML) have gained momentum as financial services firms evaluate the potential applications of AI, including for example: to enhance the customer experience and garner operational efficiencies. AIRS is committed to furthering this dialogue and has drafted the following overview addressing the use of AI within financial services, discussing AI implementation and the corresponding potential risks firms may wish to consider in formulating their AI strategy. Our hope is to contribute to and establish an industry-wide view of the potential risks and mitigants in this rapidly evolving domain of AI/ML, as they may apply to individual firms depending on their use of AI systems.
In this document, AIRS members present their views, guidance, and a structure for conceiving AI/ML risks and governance, drawing upon our combined experience implementing and managing technology risks in the financial sector. The views expressed in this paper are meant to assist individuals and organizations facing risks and governance challenges presented by AI/ML. However, it is critical that each institution assess its own AI uses, risk profile and risk tolerance and design governance frameworks that fit their unique circumstances. As such, this paper is not meant to be comprehensive or prescriptive.
1.2 AIRS
AIRS is an informal group of practitioners and academics from varied backgrounds, including technology risk, information security, legal, privacy, architects, model risk management, and others working for financial, technology organizations, and academic institutions. The AIRS working group, based in New York, was initiated in early 2019 and has grown to nearly 40 members from dozens of institutions (and continues to grow).
The AIRS Working Group seeks to promote, educate, and advance AI/ML governance for the financial services industry by focusing on risk identification, categorization, and mitigation.
1.3 Definitions & Assumptions
We use several terms throughout this document specific to AI/ML, some of which are subject to vigorous discussions and debates in the research community. Because this document attempts to form a starting point for broader AI/ML governance and risk management efforts, we purposely leverage and encourage readers to refer to various papers for the definition of AI. As such, we note that specific definitions should be (and generally are) tailored to each organization depending on the scope, risk appetite, internal structure, culture, and implementation details of AI/ML efforts.
While there is no universally accepted definition of AI, it is generally understood to refer to “a branch of computer science dealing with the simulation of intelligent behavior in computers, or the capability of a machine to imitate intelligent human behavior.” Generally, machine learning is referred to as, “a field of computer science that uses algorithms to process large amounts of data and learn from it.”[2] The term AI is broadly used and typically includes aspects of machine learning and natural language processing. For purposes of this paper, the focus is largely on the use of and potential risks related to machine learning, though the overarching discussion applies more broadly to the abovementioned areas.
1.4 A Brief Note on AI/ML Uses and Benefits
The use of AI in financial institutions is increasing as technological barriers have fallen and its benefits and potential risks have become clearer. The Financial Stability Board recently highlighted four areas where AI could impact banking specifically.[3] First, customer-facing uses could expand access to credit and other financial services. For example, combining expanded consumer data sets with new ML algorithms to assess credit quality or price insurance policies, and using AI to offer new and innovative channels to deliver financial services could be a potent way to advance financial inclusion. Also, the use of AI chatbots could provide help and even financial advice to consumers, saving them time they might otherwise waste while waiting to speak with a live operator. Second, there is the potential for strengthening back-office operations, such as advanced models for capital optimization, model risk management, stress testing, and market impact analysis. Third, AI approaches could be applied to trading and investment strategies, from identifying new signals on price movements to using past trading behavior to anticipate a client’s next order. Finally, there are likely to be AI advancements in compliance and risk mitigation by banks. AI solutions are already being used by some firms in areas like fraud detection, capital optimization, and portfolio management.
Several papers and articles describe the potential uses and benefits of AI adoption and innovation in financial services. Widespread AI/ML adoption within the financial services industry could provide a unique opportunity to significantly improve financial outcomes for consumers and businesses, if this is done responsibly. That is why AIRS has chosen to focus this paper on understanding and managing the potential risks of AI/ML so that those benefits may be realized.
2. AI Risks and Risk Categorization
Key potential risks of AI relate to potential harms that may affect organizations, consumers, or create broader detrimental effects on society. Such potential risks may arise in whole or in part from sources including the data used to train the AI system; potential risks arising from the AI system itself; potential risks arising from the usage of the AI system; and potential risks arising from poor overall governance of the AI system.
2.1 Risk Categorization
Various research papers, articles, and discussions have covered the topics of risks associated with AI. It is up to individual financial services firms to categorize potential risks of using AI: however, we have included a suggested approach to AI risk categorization in the financial services industry below.
The areas of data related risks, AI/ML attacks, testing and trust, as well as people risk constitute potential areas of risk, which could be subcategorized as illustrated in Figure 1. We address these sub-categories in further detail below.
It’s important to note that the applicability and relevance of risks illustrated in Figure 1 are dependent on an individual organization’s risk profile, appetite, and existing controls, and it is up to each firm to determine whether their existing controls are sufficient.
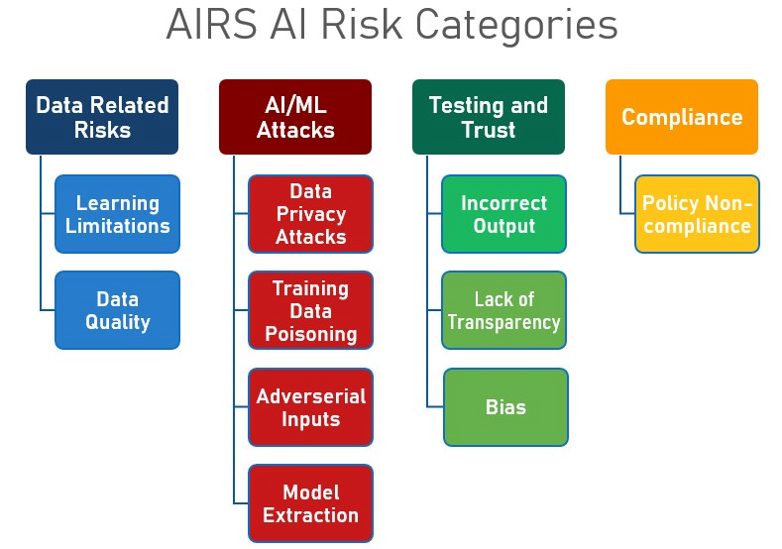
Figure 1: AIRS AI Risk Categorization
2.1.1 Inadequate Governance
Learning Limitations
Unlike humans, AI systems lack the judgment and context for many of the environments in which they are deployed. An AI/ML system is generally as effective as the data used to train it and the various scenarios considered while training the system. In most cases, it is not possible to train the AI system on all possible scenarios and data. Lack of context, judgment, and overall learning limitations may play a key role in informing risk-based reviews, and strategic deployment discussions.
Data Quality
The risk of poor data quality is not unique to AI, but for AI/ML systems, poor data quality could not only limit the learning capability of the system, but could also potentially negatively impact how it makes inferences and decisions in the future. Poor data quality could include incomplete data, erroneous or unsuitable data, stale data, or data used in the wrong context. Such deficiencies may give rise to potentially erroneous or poor predictions, or potentially result in a failure to achieve the intended objectives.
2.1.2 Potential AI/ML Attacks
The proliferation of research papers on AI/ML has increased significantly over the last decade, with many of these devoted to potential security weaknesses in AI/ML systems. Most of the known potential attacks against AI/ML systems could be grouped into one of the following categories: data privacy, data poisoning, and model extraction.
The likelihood and impact of various potential attacks are specific to each organization’s risk posture and controls. It is possible that some potential attacks illustrated below may not be relevant to a particular organization and may be mitigated by customary security controls.
Data Privacy Attacks
In data privacy attacks, an attacker is potentially able to infer the data set used to train the model, thereby potentially compromising the privacy of the data. An adversary could potentially infer sensitive information from the training data set by analyzing the parameters or querying the model. Two major attack types in data privacy include membership inference and model inversion attacks.
In a membership inference attack, an attacker could potentially determine if a particular record (or set of records) exists in a training data set. Generally, if the attack is successful, an attacker could determine, to a certain degree of probability, whether a particular record was part of the training data set used to train the AI system. In model inversion attacks, an attacker could potentially extract the training data used to train the model directly.
Training Data Poisoning
Data poisoning is the contamination of data used to train the AI/ML system, negatively affecting its learning process or output. Data poisoning could potentially be used to increase the error rate of the AI/ML system or to potentially influence the retraining process. Some of the attacks in this category are known as “label-flipping” and “frog-boil” attacks.
Adversarial Inputs
AI systems that use input from external system(s)/ user(s), interpret the input and perform an action, like classifying the input data. An adversary could potentially use a malicious input or a payload explicitly designed to bypass AI systems classifier. Such malicious inputs are known as adversarial inputs.
Model Extraction
In this attack, an adversary tries to steal the model itself. Model extraction attacks are potentially the most impactful types of AI/ML attacks, as the stolen model could be used as a ‘tool’ to create additional risks. Research into such attacks indicates that, given unlimited ability to query the model, extraction could occur without requiring high levels of technical sophistication and could be accomplished at high speeds.
2.1.3 Testing and Trust
Depending on the implementation and use case, the AI system could potentially evolve over time at varying degrees. Some forms of AI could generate complexities that may accrue, evolve or worsen over time.[4] ML models may be sensitive to environmental developments, for example, that could potentially alter their performance, Some AI systems may not have exposures to the below potential risks, either due to the nature of implementation or controls in place. Potential concerns related to testing and trust risk are discussed in detail below:
Incorrect Output
Testing and validation of AI/ML systems may pose challenges relative to traditional systems as certain AI/ML systems are inherently dynamic, apt to change over time, and by extension, may result in changes to their outputs. Testing for all scenarios, permutations and combinations of available data may not be possible, thus leading to potential gaps in coverage. The severity of these gaps may vary with each system and its applications.
Lack of Transparency
As an emerging technology, the awareness of (and hype related to) AI and the lack of adequate understanding of the technology could potentially give rise to trust issues with AI systems. There is a perception, for example, that AI systems are a “black box” and therefore cannot be explained. (We address this belief further in Section 4.) Generally, it is difficult to thoroughly assess systems that cannot easily be understood.
Bias
AI systems could potentially amplify risks relating to unfairly biased outcomes or discrimination. For example, the subjects of data ethics, fairness and the possibility of unfairly biased outcomes from the use of AI are still evolving. It is evident, however, that, depending on the use case, there is a risk that AI systems could potentially lead to unfairly biased outcomes for individuals and/or organizations. Furthermore, AI-driven unfairly biased outcomes could have privacy compliance implications, constitute regulatory, litigation and reputational risk, impact operations and result in customer dissatisfaction and attrition. Section 4 of this paper (focused on transparency, explainability and bias) discusses unfairly biased outcomes and discrimination in AI in greater detail.
2.1.4 Compliance
Policy Non-Compliance
As AI implementations mature in organizations, their impact on existing internal policies should be considered. Regulatory bodies have expressed growing interest in AI deployments in the financial industry. Regulators have formed working groups representing various authorities across the globe to discuss supervisory challenges posed by emerging technologies, which have led to the publication of guidelines, white papers, and surveys. This interest is driven by the understanding that AI/ML poses new challenges, and readers should evaluate how regulations may impact the use and governance of AI/ML. AIRS is not advocating for new regulation(s), but merely would encourage readers to monitor existing regulations and their potential applicability to AI.
3. AI Governance
3.1 The AI Governance Survey
To better understand current enterprise-wide governance practices within the industry, AIRS members were surveyed about their approach to managing AI/ML risk. Figure 2 depicts the results of the survey.
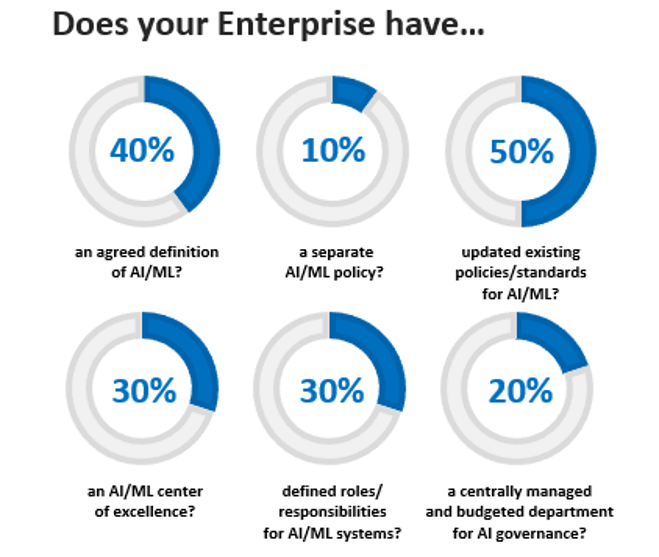
Figure 2: AIRS AI Governance Survey
Given the results of this survey, which shows that the financial services industry is focused on AI, and potentially in early stages of adoption, the industry would benefit from a common set of definitions and more collaboration in developing risk categorization and taxonomies.[5] The response to the questions do not reflect future plans of the institutions. For example, “updated existing policies/standards for AI/ML?” merely confirms if a policy was updated and does not necessarily explain if the respondents will or plan to update their policies; one potential reason could be that the firms believe that existing controls address the potential AI risks.
3.2 AI Governance
As AI use advances and becomes more widespread within the industry, AIRS acknowledges that there are multiple ways to govern potential risks and any risk management framework should be tailored to each individual firm’s unique circumstances. The following are just a few examples of what some firms may find useful as they think about managing risks related to their own adoption of AI systems. For instance, some firms may find it more effective to adapt their existing risk infrastructure to manage AI risk rather than adding entirely new structures.
Four core components of AI governance include: definitions, inventory, policy/standards, and framework, including controls.
3.2.1 Definitions
Depending on the adoption, environment, and culture of an organization, there may be a long series of nuanced definitions for AI/ML. As the first step to achieving AI governance, a clear definition of what constitutes AI (and what does not) is critical for any organization. This definition provides the foundation and establishes a clear understanding of the other components of the governance structure, informing the remaining building blocks that comprise the overall AI program (e.g., inventory).
Any definition of AI should consider, among other factors, the variety of techniques used by the organization in training and developing the AI, what distinguishes AI from other traditional rule-based systems, and the implications of the definition, enabling the kind of AI inventory efforts we set forth below. Definitions and supporting documentation should provide clarity related to how various stakeholders – including Senior Management, Legal, System Developers, Compliance, and Information Security Officers – identify with the AI definition relative to other well-established definitions.[6]
3.2.2 Inventory
The purpose of an inventory is to allow the organization to identify and track the AI/ML systems it has deployed and monitor associated risk(s) (if any). Such an inventory might describe the purpose for which the system is designed, its intended use, and any restrictions on such use. Inventories might also list key data elements for each AI/ML system, including any feeder systems/models, the owners, developers, validators, and key dates associated with the AI/ML lifecycle.
Organizations may benefit from the implementation of protocols, structures, frameworks, and tools to assist in maintaining an accurate and comprehensive systems inventory.
3.2.3 Policies
Existing policies and standards might already apply to many use cases for AI. In such circumstances, however, additional or revisions to policies and standards may be necessary to ensure that AI is deployed appropriately. Potential enhancement of existing processes and the creation of new documentation should, as a result, be considered.
We note that ethical principles for AI have been in discussion for some time in the industry, with a handful of institutions circulating these AI ethical principles publicly. Members of the AIRS group have seen firsthand the positive impact these principles could have, and actively encourage their further development, including as appropriate in conjunction with any data governance efforts regarding ethical use of data.
3.2.4 Framework
AI governance frameworks could help organizations learn, govern, monitor, and mature AI adoption. An AI governance framework might begin with an organization identifying key stakeholders representing various groups and departments. Such a ‘coalition’ may be formalized in a Center of Excellence (CoE), working group, or council, among other examples. Such groups might develop best practices for their organization, share knowledge, and build guard rails for the use of AI systems. These efforts are generally most successful when they establish close links with technology, data engineers and line of business stakeholders to complement existing frameworks, and to support existing workflows in monitoring and oversight of the activities of AI systems and AI-enabled products.
In reviewing an AI-enabled initiative, the ‘coalition’ should take various considerations into account, (as applicable) including data ethics, privacy rights, applicable regulatory considerations, whether the data on which the AI system is being trained is suitable (i.e., was it provided for this purpose or is it being leveraged in a manner unrelated to that for which it was provided), whether notice of such use may be required to third parties, whether the data set is appropriately safeguarded (via access right controls and encryption protocols, for instance), and the manner of supervisory oversight that is appropriate to evidence control over the AI system, whether developed internally or by a third party.
Depending on the scale of adoption, a formal approval process might be put in place, governed by a central body having subject matter expertise from various fields. When sufficient comfort with AI governance is achieved, this central structure may also be disbanded and replaced with a federated structure that could cater to business-specific needs and risks.
Note that identification of potential AI/ML risks (as set forth in Section 2) is critical to formulating an operational risk and control framework. Upon identification of potential risks, a gap analysis might then be instituted against existing controls. Depending on the control library of an institution, this may require participation from multiple control owners and requires a structured approach and thorough planning. Results of the gap analysis should then lead to the creation of potential new or enhanced controls to mitigate against the identified potential AI/ML risks.
AI governance frameworks should also consider a host of other factors, some of which we outline below.
Monitoring and Oversight
A central monitoring and escalation process is, in many cases, essential: providing sufficient exposure within an organization to the decisions being made and an opportunity to raise concerns or challenges when appropriate. This structure should enable the monitoring system to adapt to the changing needs of the organization, as AI adoption matures or substantial changes in the industry occur. It’s noted that some firms may believe that existing monitoring and oversight procedures sufficiently address potential AI risks.
Existing governance systems in most organizations are designed for processes where there is a high degree of human involvement. Business experts, for example, are oftentimes on-hand to override erroneous results. Reducing or removing interventions may, however, improve the accuracy, consistency, and efficiency of existing processes. This is especially true where each new data iteration dynamically optimizes the AI system and improves upon it – a chatbot, for example, learning and tuning its response with each customer interaction. Governance around these dynamically calibrating processes typically require additional safety protocols, including, for example, more robust and continuous monitoring, pre-defined performance thresholds, and “kill-switches” that could remove the system from deployment entirely, if necessary, depending on the use case.
Third-Party Risk Management
The use of AI/ML deployment may involve third party applications and/or data, as discussed in Section 2, which could enable scalability, increased compute power and access to vendors that are part of the larger fintech ecosystem. As a result, firms may need to strengthen their third-party risk management (TPRM) capabilities. These developments may test certain aspects of current practices, such as TPRM transparency around model interpretability, information security issues for cloud-based service providers, and broader concerns around technology dependencies for the third parties themselves. Depending on the use case, Firms may consider including contractual clauses for third parties regarding the AI system’s testing methodology, explainability of the results generated by the system, and/or intellectual property rights which may be derived from use of the system.
Three Lines of Defense
Most financial institutions follow a three-lines-of-defense model, which separates front line groups, which are generally accountable for business risks (the First Line), from other risk oversight and independent challenge groups (the Second Line) and assurance (the Third Line). AI governance frameworks should ensure that sufficient oversight, challenge, and assurance requirements are met in AI system development and utilization. Furthermore, as both the potential risks and regulations related to AI are evolving, the second and third lines of defense should, likewise, ensure they have adequate subject matter expertise to effectively challenge the first line in evaluating the proposed use and implementation of the AI systems, as outlined earlier in Section 2.
Roles and Responsibilities
Every organization is different with respect to their internal organizational structure and general roles and responsibilities. The roles/activities below provide some examples for organizations that are discussing roles and responsibilities with respect to AI implementations to consider. It is not intended to be an exhaustive or prescriptive list.
Ethics Review Board
An ethics review board may review AI projects in accordance with an organization’s ethical principles, e.g. AI deemed to be high risk.
Center of Excellence
A Center of Excellence (CoE) may provide a knowledge-sharing platform in an organization. Depending on the organization, a CoE could create a collective view and create and share best practices. Furthermore, the CoE could maintain engagement with the industry to share and learn best practices.
Data Science
Some organizations have mature Data Science practices. In addition to their assigned responsibilities, the Data Science team could manage AI system inventory and version control.
ML Operations
A ML operations team provisions data for analysis by the data science team. They may also create and maintain data sets for the purpose of training AI systems.
4. Interpretability and Discrimination
Interpretability (presenting the AI system’s results in human understandable format), and discrimination (unfairly biased outcomes) are crucial concepts that factor into the risks associated with AI/ML systems used for certain use cases. In this section, we explore potential risks associated with discrimination and interpretability as they relate to certain applications of AI, e.g., loan approvals.
4.1 Discrimination in AI
Depending on the use case, AI may potentially lead to discriminatory and/or unfairly biased outcomes if not implemented appropriately. Poor implementation may arise from biased data, the AI system itself not being properly trained or when there are alternate systems and data sources that could potentially be used to generate better outcomes for disadvantaged groups. Ultimately, the use of an AI system which may cause potentially unfair biased outcomes may lead to regulatory non-compliance issues, potential lawsuits and reputational risk. That said, these risks could be managed. There is even growing evidence that AI/ML systems could be harnessed to more effectively control for discriminatory outcomes.
Existing Legal and Regulatory Frameworks
Federal and state statutes prohibit discrimination in areas that impact our daily lives, including employment, housing, and lending, to name a few. By way of example, a potential impact in the use of AI for lending is described in greater detail below.
The primary U.S. federal statutes that define illegal discrimination in lending are the Equal Credit Opportunity Act (ECOA) and the Fair Housing Act (FHA); however, lenders are subject to many other federal regulations and state laws addressing fairness. Each statute defines types of “protected classes,” such as gender, race, or ethnicity, that a lender cannot legally disfavor.
Generally speaking, three types of discrimination are recognized by federal banking regulators: overt discrimination, disparate treatment, and disparate impact when not supported by a legitimate business justification. Disparate treatment discrimination could occur when similarly situated individuals are treated differently based on a prohibited basis, but the treatment does not have to be motivated by prejudice or an intent to discriminate. In an AI context, this may potentially occur, for example, when a firm explicitly uses protected class status in an AI system used to underwrite creditworthiness.
Disparate impact, on the other hand, occurs when a system includes features that lead to disproportionately unfavorable outcomes for a protected class. Importantly, evidence of disparate impact is almost always assessed independently of the accuracy and validity of the system. In other words, just because a given system is statistically sound does not mean that it is legally non-discriminatory. Such systems are generally not considered legally discriminatory if they and their constituent features could be demonstrated to meet a legitimate business need and where no less discriminatory alternative system or process could be identified that also meets those needs.
Concerns over using and potentially amplifying implicitly biased data also arise in other contexts. For instance, the New York Department of Financial Security (NY DFS) discussed [7] the use of external consumer data and information sources in insurance underwriting, noting the potential of leveraging these sources to help establish lifestyle indicators that may inform the review of an application for insurance coverage. In doing so, however, NY DFS observed that such data may be inaccurate or unreliable, and its use may result in a significant detrimental impact to the insured.
Similarly, in a speech [8] by Charles Randell, Chair of the UK Financial Conduct Authority, concerns over misuse of big data to inform potentially detrimental outcomes were raised, with a real-world example in the use of data mining credit card charges for services such as marriage counseling, and reducing cardholders’ credit limits on the basis of the correlation between marriage breakdown and debt default. The use and potential for misuse of big data is no longer a theoretical concern and should be considered in determining the types of data that may be used in developing AI/ML systems.
We reference these legal and regulatory considerations to illustrate existing standards that already apply to many algorithmic activities of financial institutions, especially as they relate to unfairly biased outcomes.
Data as a Cause of Discriminatory AI
A host of factors may result in AI-related illegal discrimination. Input data may cause illegal discrimination if it identifies or closely proxies class membership, if it causes protected class members to experience less favorable outcomes, or if it is differentially predictive of the outcome for the protected class.
Traditional data inputs, such as many credit bureau attributes, tend to be less likely to raise disparate impact concerns because they are generally thoroughly vetted and accepted for credit worthiness. They may also be differentially predictive if the system’s weights or coefficients do not properly account for class-specific idiosyncrasies.
Non-traditional data, such as utility payment history, rental payments, or a person’s digital footprint (including social media posting), may generate heightened concerns relative to traditional data. From a fairness perspective, such data may have substantial merit, as its use has been shown to expand access to the financial system for unbanked or underserved populations that are often more likely to be members of some protected groups. However, such data use often raises coverage and accuracy concerns.
Algorithms as a Cause of Discriminatory AI
Algorithms themselves may result in discriminatory outcomes exacerbated by their complexity and opacity. Some of this concern arises from the fact that some machine learning algorithms create variable interactions and non-linear relationships that are too complex for humans to identify and review. These relationships have the potential to cause disparate treatment discrimination by creating proxies for protected class status. To some degree, these concerns have been lessened by advances in explainable AI techniques that allow additional insight into these complex relationships, which we address in Subsection 4.2 below.
System misspecification may also cause discriminatory outcomes. Here, features may be independently predictive of both the outcome and protected class status, but the class effect is incorporated into the prediction. For example, suppose a credit system included whether a person tended to shop at a discount store. It is likely that such a variable would capture a measure of wealth, which may be a reasonable predictor of repayment, but may also unintentionally capture a race effect. In addition, if the store is more likely to be located in minority neighborhoods, then the system may further exacerbate this effect. That is, the variable may act as a proxy for the neighborhood, which in turn acts as a proxy for race. Importantly, this is not a problem that is unique to AI. In fact, to the extent that machine learning is more accurate than traditional methods, it may be more likely to identify such a relationship and remove the non-predictive race effect.
4.2 Interpretability/Explainability
Interpretability relates to the ability of humans to gain insight into the inner workings of AI systems, which may be complex and opaque. In a practical sense, the two primary aspects of AI/ML interpretability are directly interpretable system mechanisms and posthoc explanations (explainability) of system mechanisms and predictions.
Well-known interpretable systems include linear systems, decision trees, and rule-based systems, where internal system mechanisms are composed of a relatively small number of learned coefficients or Boolean rules. Examples of newer and perhaps relatively more accurate and sophisticated types of interpretable AI/ML systems include scalable Bayesian rule lists, Explainable Boosting Machines (EBMs), monotonic Gradient Boosting Machines (GBMs), various Bayesian or constrained variants of traditional AI/ML systems or other novel interpretable-by-design systems.[9]
Inconsistent Explanations
Unlike more traditional linear systems, the same training data set may be used to train many possible accurate AI/ML systems, such that any AI/ML system a practitioner trains is just one of many potentially good systems. As a result, while the outcome of the AI/ML systems may be similar, there may be many different logical explanations for how the AI generated the output. Therefore, two systems giving different explanations for the same result or decision may create unwanted outcomes. Explanation inconsistency could also rear its head when systems are refreshed. When using low quality or inconsistent explanation techniques, simply retraining a system on newer data could also result in different explanations for the same customer and decision.
Posthoc explanation methods, such as feature importance and partial dependence, give approximate summaries of AI/ML system mechanisms or predictions across an entire dataset. Many newer explanation approaches tend to focus on high-fidelity summaries of local system behavior, essentially attempting to describe why an AI/ML system made a decision about a single customer, transaction, or other entity. These newer techniques include local interpretable system-agnostic explanations (LIME), Shapley additive explanations (SHAP), or saliency maps. Importantly, novel interpretable systems and posthoc explanations are already in use today.[10]
Methods for interpretability facilitate the human understanding of AI/ML systems, which could help to mitigate many of the risks elaborated throughout this paper. Such interpretability could help mitigate the risks from incorrect AI/ML system decisions, enable security audits of AI/ML systems, and align with regulatory compliance efforts.
Detection and Appeal of Incorrect Decisions
Because AI/ML systems are probabilistic, they may make incorrect decisions. In extremely opaque systems, however, neither the developer nor the user may have enough insight to understand how, or even if, the decision is wrong. This fact makes interpretability of high-impact AI/ML decisions a significant imperative and a source of potential risk. If such effects are adverse or otherwise perceived as incorrect, both organizations and impacted individuals alike may seek to detect and mitigate the harms created by the AI/ML-based decisions.
Security Audit
Malicious actors could potentially misuse or abuse traditional IT systems in multiple ways, and AI/ML is no exception. Indeed, security is evolving in the world of AI/ML, and interpretability plays a major role in ensuring that such systems are protected. Red team or white-hat hacking audits or exercises to test AI/ML systems may, for example, use variants of posthoc explanation techniques in system stealing, system inversion, and membership inference attacks against AI/ML systems.
Regulatory Compliance
Interpretable systems, posthoc explanations, and the documentation they facilitate may also be required under several applicable regulations and legal frameworks, such as the Equal Credit Opportunity Act, the Fair Credit Reporting Act, and the E.U. General Data Privacy Regulation (GDPR), among others. This both increases the importance of interpretability in AI/ML systems generally and highlights the compliance-related risks associated with their use.
5. Common Practices to Mitigate AI Risk
In this section, we outline potential mitigants and emerging best practices that could guide firms in their internal discussions regarding potential AI risks. These insights are based on our collective experience, and the suggestions we outline are, as a result, not meant to be comprehensive or prescriptive.
In general, machine learning pipelines contain three possible points of intervention: the training data, the learning procedure, and the output predictions, with three corresponding classes of mitigation algorithms – pre-processing, in-processing, and post-processing.[11] The advantages of post-processing approaches are that they may not require access to the training process and are thus suitable for run-time environments. Moreover, post-processing algorithms operate in a black-box approach, meaning that they do not necessarily need access to the internals of models, their derivatives, and may therefore be applicable to any machine learning model (or amalgamation of models).
5.1 Oversight and Monitoring
Oversight Processes
An oversight process based on thorough monitoring to validate the outputs, thresholds, and other aspects of the system could help maintain the overall accuracy and efficiency of AI systems. Oversight processes might begin with the creation of an inventory of all AI systems employed at the organization, the specific uses of such systems, techniques used, names of the developers/teams and business owners, and risk ratings – measuring, for example, the potential social or financial risks that may come into play should such a system fail. Another process might also evaluate the inputs, and the outputs of the AI system, as well as the AI system itself. Even though data quality requirements are not specific to AI/ML, data quality has significant impact on AI systems, which learn using data and provide output based on that learning. Training data could be assessed for data quality as well as for potential biases the data set may contain. AI system evaluation could involve benchmarking against alternative models and applying known techniques to enable model interpretability, where applicable and feasible. Understanding the factors driving AI systems recommendations could improve trust in the AI systems.
Monitoring for Drift
Drift may lead to multiple types of errors and risks in AI systems. Poor model accuracy, for example, could sometimes be attributed to the relationship between target variables and independent variables changing with time. As such, drift detection could play an important capability in mitigating some types of AI-related risks, including characteristics that contribute to a model’s security, privacy, and fairness. Monitoring could account for the data received by the model in production and estimate the accuracy of the model, which is one of the ways to provide insight into the “accuracy drift” of the model. Monitoring could also assess if input data significantly deviates from the model’s training data, which could help inform the identification of “data drift.”
Detecting accuracy drift may be helpful to enterprise applications in that it may identify a decrease in model accuracy before the change results in a significant impact to the business. Accuracy drift can make your model worse. Data drift, on the other hand, helps enterprises understand the change in data characteristics at runtime.
5.2 Addressing Discrimination in AI
Most lending institutions employ compliance, fair lending, and system governance teams that review input variables and systems for evidence of discrimination. Eventually, some or even most of this work may be automated and streamlined through technological advances and the use of de-biasing AI (discussed below). Fair AI, nevertheless, may require a human-centric approach. It is unlikely that an automated process could fully replace the generalized knowledge and experience of a well-trained and diverse group reviewing AI systems for potential discrimination bias. Thus, the first line of defense against discriminatory AI typically could include some degree of manual review.
Some recently researched algorithms that diminish discrimination have also been shown to minimize class-control disparities while maintaining the system’s predictive quality. Mitigation algorithms find the “optimal” system for a given level of quality and discrimination measure in order to minimize these disparities. In this, the algorithms attempt to find alternative systems where, for any given level of discrimination, no system can be found with a higher level of quality. Conversely, for any given level of quality, no system can be found that decreases discrimination. Further testing and research need to happen before leveraging such algorithms in a production environment.
Broadly, these methods could be separated into two groups: more traditional methods that search across possible algorithmic and feature specifications in order to find less discriminatory but valid systems, and more recently developed approaches that change the input data or the optimization functions of the algorithms themselves.[12]
Minimizing disparate impact may focus on feature selection whereby typically one or two variables that drive disparate impact are excluded from the system, while a few other variables are tested as replacements. These methods have been shown to have limited success in complex AI/ML systems.
More recently developed approaches minimize discrimination by focusing on data pre-processing, within-algorithm decision making, and output post-processing.[13] Whether these methods are suitable for use in a particular case depends on the legal environment in which the system is used and the system’s usage itself.
5.3 Enhancing Interpretability and Explainability
This section focuses on AI use cases where interpretability/explainability is required by law or is otherwise appropriate. AIRS acknowledges that it may not always be necessary or appropriate. As of this writing, there is no commonly agreed upon standard definition of AI explainability.
Ensuring Quality Explanations
Ensuring that AI/ML explanations (explainability) are both reliable and useful could be a challenge for many organizations. Like the underlying AI/ML systems, for example, AI/ML explanations could be rough approximations, inaccurate, or inconsistent. Inconsistency bears special consideration in financial services, especially in the context of adverse action notices for credit lending decisions. (Posthoc explanation techniques are receiving considerable attention for the generation of adverse action notices. However, a thorough discussion of explanation in the adverse action notice context is outside the scope of this broad report.[14])
Depending on specific implementations, organizations may test explanatory techniques in human evaluation studies or, for accuracy and stability, on simulated data, to potentially reduce risks associated with explainability.
5.4 Potential Risk Mitigation
Recent research indicates that providing explanations of how AI systems work, along with predictions, could help malicious actors. To mitigate the potential risks, organizations should only share the minimal information required by respective consumers or as applicable by law. Depending on the implementation and control environment, AI/ML systems trained on sensitive data with predictions accessible to end-users could also be protected using existing security measures, such as real-time anomaly detection, user authentication, and API throttling.[15]
Traditional strong technology and cyber controls could act as effective risk mitigants for AI implementations. The evolving field of adversarial learning may help with building secure machine learning systems as it matures. Although this is still a field of evolving research, some theoretical mitigation techniques are being further researched in the technology industry. For example, one suggested method for maintaining the privacy of the training data is differential privacy. Differential privacy makes data anonymous by introducing random noise to a dataset, which allows for statistical analysis without any personal information being identifiable. Therefore, the results of the system are similar even if a particular user/data element record is omitted. Although mitigation techniques are still being researched for AI/ML attacks discussed in Section 2, depending on implementations and environment, having strong technology and cyber controls could act as effective mitigation. Prevention of model extraction attacks could potentially be achieved using strong information security practices; however, the identification of an extracted model is possible with a method known as watermarking. In watermarking, the AI/ML system is trained to produce unique outputs for certain inputs. If another system produces the same unique output for the same inputs, it may point to Intellectual Property theft.
6. Acknowledgments
For any inquiries or questions regarding AIRS or the white paper, please contact Yogesh Mudgal (yogesh@airsgroup.ai).
AIRS would like to thank our authors for their key role in the creation and development of the AI/ML Risk and Governance white paper.
Yogesh Mudgal, AIRS Founder/Curator; Operational Risk Management, Citi
Andrew Burt, Managing Partner at bnh.ai, Chief Legal Officer at Immuta
Ruchir Puri, Chief Scientist, IBM Research, IBM Fellow at IBM
Patrick Hall, Advisor at H2O.ai, Principal Scientist at bnh.ai, Visiting Professor at GWU
Nicholas Schmidt, Director and AI Practice Leader at BLDS, LLC
Anusha Dandapani, United Nations International Computing Centre, Adjunct Faculty at NYU
Anuj Prakash, Model Risk Management, HSBC
Nick Lewins, Financial Services Lead at Microsoft Research
Armando Lemos, Technology Risk at Wells Fargo
Brennan Lodge, Data Science Lead at Goldman Sachs
Kartik Hosanagar, John C. Hower Professor at The Wharton School, Director of Wharton AI for Business
Marina Kaganovich, Director, U.S. CIB Digital Advisory Compliance, BNP Paribas
AIRS would like to thank the following contributors for their valuable insights and contribution to the AI/ML Risk and Governance white paper (in alphabetic order)
Akash Verma, Discover
Andres Fortino, PhD, NYU
Bruno Domingues, Intel
Daragh Morrissey, Microsoft
Kawbena Poku, Discover
Kevin Fitzpatrick, Wells Fargo
Keyvan Kasiri, Bank of Montreal
Kjersten Moody, State Farm
Manan N. Rawal, MUFG
Meeta Dash, Appen
Narahara (Chari) Dingari, PhD, DeutscheBank
Parviz Peiravi, Intel
Patrick Dutton, HSBC
Priti Ved, Bloomberg
Contributions are made in an individual capacity and do not represent the views of the institution who the authors and contributors work for or are associated with.
[1] AIRS is an informal group of practitioners and academics from varied backgrounds, including technology risk, information security, legal, privacy, architects, model risk management, and others, working for financial and technology organizations and academic institutions. The AIRS working group, based in New York, was initiated in early 2019. It has grown beyond the original members to around 40 members from dozens of institutions.
[2] FINRA’s Report on the Use of AI in the Securities Industry: https://www.finra.org/sites/default/files/2020-06/ai-report-061020.pdf
[3] Financial Stability Board, Artificial Intelligence and Machine Learning: https://www.fsb.org/wp-content/uploads/P011117.pdf. See also, Brainard, “What Are We Learning about Artificial Intelligence in Financial Services? https://www.federalreserve.gov/newsevents/speech/files/brainard20181113a.pdf
[4] See D. Sculley et al., “Machine Learning: The High Interest Credit Card of Technical Debt,” SE4ML: Software Engineering for Machine Learning (NIPS 2014 Workshop), available at https://research.google/pubs/pub43146/.
[5] Our results appear to align with broader public industry surveys and trends as well. See, for example, “Transforming Paradigms: A Global AI in Financial Services Survey,” World Economic Forum, January 2020, available at http://www3.weforum.org/docs/WEF_AI_in_Financial_Services_Survey.pdf.
[6] See, for example, SR 11-7 Guidance on Model Risk Management SR 11-7, April 2011, available at https://www.federalreserve.gov/supervisionreg/srletters/sr1107.htm.
[7] See NY DFS Insurance Circular No. 1, January 18, 2019, https://www.dfs.ny.gov/industry_guidance/circular_letters/cl2019_01
[8] See “How can we ensure that Big Data does not make us prisoners of technology?”, July 11, 2018, https://www.fca.org.uk/news/speeches/how-can-we-ensure-big-data-does-not-make-us-prisoners-technology
[9] See, for example, XGBoost, h2o’s GBM or Microsoft’s InterpretML toolkit, available at https://xgboost.readthedocs.io/en/latest/tutorials/monotonic.html, https://github.com/h2oai/h2o-3/blob/master/h2o-py/demos/H2O_tutorial_gbm_monotonicity.ipynb and https://interpret.ml/ respectively.
[10] Jie Chen, “Deep Insights into Explainability and Interpretability of Machine Learning Algorithms and Applications to Risk Management,” JSM 2019 Online, available at https://ww2.amstat.org/meetings/jsm/2019/onlineprogram/AbstractDetails.cfm?abstractid=303053.
[11] This three-part approach aligns, broadly, with the key sources of risk set forth in Subsection 2.1.
[12] For a further discussion of potential and utilized techniques that can mitigate the disparate impact in financial services, see Nicholas Schmidt and Bryce Stephens, “An Introduction to Artificial Intelligence and Solutions to the Problem of Algorithmic Discrimination,” Conference on Consumer Finance Law (CCFL) Quarterly Report, Volume 73, Number 2 (October 2019) available at https://arxiv.org/abs/1911.05755.
[13] IBM’s AI Fairness 360 toolkit and Microsoft’s FairLearn toolkit, for example, provide open-source compilations of many of the metrics and techniques that have been developed recently, available at https://github.com/IBM/AIF360 and https://fairlearn.ai respectively.
[14] For a more detailed discussion of adverse action notices and posthoc explanation see Navdeep Gill et al., “A Responsible Machine Learning Workflow with Focus on Interpretable Models, Posthoc Explanation, and Discrimination Testing,” Information 11(3), March 2020, available at https://www.mdpi.com/2078-2489/11/3/137.
[15] For a high-level discussion of attacks on AI systems and proposed mitigation tactics, see Sophie Stalla-Bourdillon et al., “Warning Signs: Identifying Privacy and Security Risks to Machine Learning Systems,” Future of Privacy Forum, September 2019, available at https://fpf.org/wp-content/uploads/2019/09/FPF_WarningSigns_Report.pdf.