Student
Hassan Hammoud, W’23
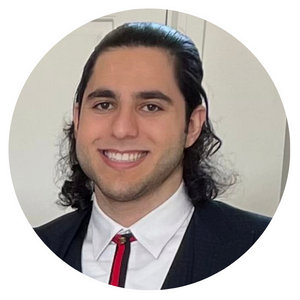
What is your area of study?
BS, Economics with concentrations in Finance and Statistics and minors in Mathematics and Data Science
MS, Engineering in Data Science
What drew you to Penn?
Honestly, the insane amount of resources available to students. As a first-generation college student from Detroit, reading about all the opportunities to get involved with real-world projects at Penn and especially Wharton was mind-blowing. Looking back, taking the step to challenge myself and move over here was probably the best decision I’ve ever made.
How are you engaged with AIAB?
I’ve been involved in a variety of AIAB initiatives, from the data science workshops in my first semester to my involvement as a Wharton Analytics Fellows board member today. I constantly check in to see what’s going on, and I love all of the resources AIAB has to offer, from rich data sources for personal projects to self-guided, online learning modules.
What were your top takeaways from working on a AIAB project?
That being committed, prepared, and willing to learn is half of the battle. I was thrown into the deep end with my first AIAB project as a freshman without the most refined analytics skills, but having the initiative to learn and absorb as much as I could from my team members and the internet helped me build my skills at high speed. You don’t need to be a genius programmer to learn data science — the trial by fire that comes with throwing yourself into a project is arguably the best way to learn!
Why is working on current datasets and actual business questions important to your educational analytics experience at Penn?
This isn’t a particularly novel insight, but data science education is very different from data science in practice. Most university courses provide you with relatively clean, siloed data such that most of your time is spent figuring out and building a machine learning model. In my experiences with AIAB projects and in my past internships, most of my time has actually been spent coming up with ways to find, load, and clean data before it can even be analyzed. Modeling is taught as the most important part of the data science pipeline, when in reality only about 10-15% of your time working on a project is going to actually be modeling-related.
How has your experience with AIAB shaped your career goals?
My internship this past summer actually came as a result of a past engagement with a firm through the Analytics Accelerator, so AIAB has already jump-started my career! Furthermore, I never knew I wanted to do data science until I joined the Wharton Analytics Fellows, and now those skills are something I will treasure for my entire life. Although I am considering a career in several industries from technology to finance, I know that I want my job to be driven by data science, no matter what.
What advice would you give students interested in starting a career in data analytics?
Don’t be afraid to fail! The biggest mistakes students make when starting out is giving up when they don’t see success right when they start programming. Even though I came into college with very limited programming experience, I was able to learn at high speed just because I was willing to try new things and fail at them, as long as I used those failures to learn more and to continue sharpening my skills.
To that end, you should definitely work on some projects to add to a “data science portfolio.” Just think about some questions that have been going through your mind and see if you can find a dataset online that’ll help you solve them! If you love the discipline and have a passion for data, these projects are a fun and easy way to build both your skills and your resume.
If you could be a statistical model, which one would it be and why?
That’s a tough one… I’d probably be one of the probabilistic models Professor Peter Fader’s discusses in his research. I took his course (STAT/MKTG 476/776) last semester and I was in awe at the elegance and beauty of some of the models we discovered. From the simple Pareto II model to his most complex CLV customer-based-corporate-valuation-bells-and-whistles model, all of them were so fascinating.