AI and the Future of Work
Sponsored by AI at Wharton
May 22-23, 2024
Jon M. Huntsman Hall
3730 Walnut Street, 8th Floor
Philadelphia, PA 19104
Presenters
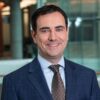
Laurence Ales, Professor of Economics, Tepper School, Carnegie Mellon University
Title: “AIdiocracy”
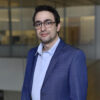
David Almog, PhD student, Kellogg School of Management, Northwestern University
Title: “AI Oversight and Human Mistakes: Evidence from Centre Court”
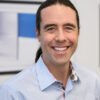
Matt Beane, Assistant Professor Technology Management, UC Santa Barbara
Title: “Engineering Skill: The Co-Development of AI-Enabled Robots and Career-enhancing Nonprofessional Skill at JointBot”
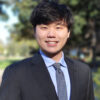
Sungwoo Cho, PhD student, UCLA
Title: “The Effect of Robot Assistance on Skills“
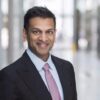
Anil Doshi, Assistant Professor, UCL School of Management (London)
Title: “How Generative AI Affects the Workplace Perceptions and Behavior”
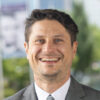
Lukasz Drozd, Economist and Advisor, Federal Reserve Bank, Philadelphia
Title: “Understanding Growth Through Automation: The Neoclassical Perspective”
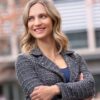
Anastassia Fedyk, Assistant Professor of Finance, Haas School of Business, University of California-Berkeley
Title: “Artificial Intelligence and Firms’ Systematic Risk”
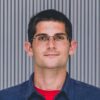
Morgan Frank, Assistant Professor, Department of Informatics and Networked Systems, University of Pittsburgh
Title: “AI exposure predicts unemployment risk“
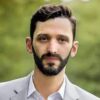
Nicholas Otis, PhD Candidate, Berkeley Haas
Title: “The Uneven Impact of Generative AI on Entrepreneurial Performance”
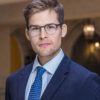
Anders Humlum, Assistant Professor of Economics, Booth School of Business, University of Chicago
Title: “The Adoption of ChatGPT”
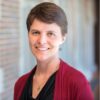
Sonia Jaffe, Principal Researcher, Microsoft
Title: “The impact of generative AI on collaboration at work”
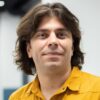
Alireza Javadian Sabet, PhD Student, School of Computing and Information, University of Pittsburgh
Title: “Quantifying exposure to Large Language Models in millions of college syllabi”
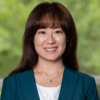
Xi Kang, Assistant Professor, Owen Graduate School of Business, Vanderbilt University
Title: “Machine Predictions and Causal Explanations: Evidence from a Field Experiment”
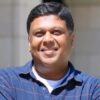
Arvind Karunakaran, Assistant Professor of Management Science & Engineering, Stanford University
Title: “Role Redesign in the Wake of Generative AI within Organizations: Paralegals and Junior Attorneys in a Corporate Law Firm”
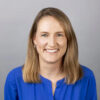
Katherine Kellogg, Professor of Work and Organization Studies, MIT Sloan School of Management
Title: “Don’t Expect Tech Savvy Juniors to Teach Senior Professionals to Effectively Use Generative AI”
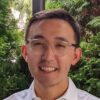
Kevin Lee, PhD student, University of Chicago, Booth School of Business
Title: “Value Aligned Large Language Models“
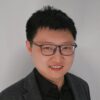
Frank Li, Assistant Professor, Sauder School of Business, University of British Columbia
Title: “Jump Starting the AI Engine: The Complementary Role of Data and Management Practices”
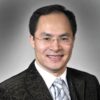
Xueming Luo, Professor, Fox School, Temple University
Title: “From Overwhelmed to Empowered: How Artificial Intelligence Augments Transformational Managers in Employee Training at the Workplace”
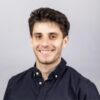
Benjamin Manning, PhD student, Sloan School of Management, MIT
Title: “Automated Social Science: A Structural Causal Model-Based Approach“
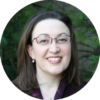
Kristina McElheran, Assistant Professor, University of Toronto
Title: “Digitization, Analytics, and AI in U.S. Manufacturing”
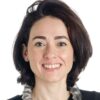
Marina Mendes Tavares, Background Economist, International Monetary Fund
Title: “Gen-AI: Artificial Intelligence and the Future of Work“
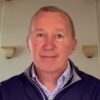
Tom Mitchell, Founders University Professor, Machine Learning Department, Carnegie Mellon University
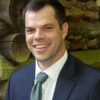
Alex Moehring, PhD student, MIT Sloan School of Management
Title: “Combining Human Expertise with Artificial Intelligence: Experimental Evidence from Radiology“
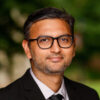
Madhur Mohar, PhD student, University of Georgia
Title: “The Impact of Generative AI on Creative Suppliers’ Product and Pricing Decisions”
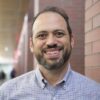
Ethan Mollick, Ralph J. Roberts Distinguished Faculty Scholar; Associate Professor of Management;
Academic Director, Wharton Interactive, The Wharton School, University of Pennsylvania
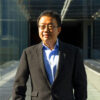
Min-Seok Pang, Professor, University of Wisconsin-Madison
Title: “Automated Enforcement and Traffic Safety”
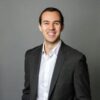
Nicholas Pangakis, PhD Candidate, University of Pennsylvania
Title: “Automated Data Labeling with Generative AI”
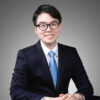
Peter S. Park, MIT AI Existential Safety Postdoctoral Fellow (Tegmark Lab)
Title: “AI-Augmented Predictions: LLM Assistants Improve Human Forecasting Accuracy“
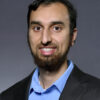
Hatim Rahman, Assistant Professor Kellogg School of Management, Northwestern University
Title: “Beyond Data Collection: Examining Artificial Intelligence Data Creation in Organizations”
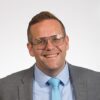
William Resh, C. C. Crawford Professorship in Management and Performance, Sol Price School of Public Policy, University of Southern California
Title: “Complementarity, Vulnerability, and Replacement: Artificial Intelligence in the United States Federal Labor Market”
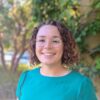
Emma Rockall, PhD Candidate, Department of Economics, Stanford University
Title: “AI Adoption and Inequality“
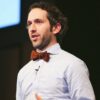
David Rothschild, Senior Principal Researcher, Microsoft Research
Title: “Math Education With LLMs: Peril or Promise?“
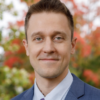
Gregor Schubert, Assistant Professor of Finance, Anderson School of Management, UCLA
Title: “The Labor Impact of Generative AI on Firm Value“
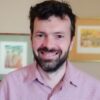
Antonios Stamatogiannakis, Assistant Professor of Marketing, IE Business School, IE University
Title: “A Warning for AI Biases at Work: Evidence from 40,000 Conversations with Large Language Models“
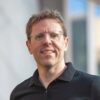
Neil Thompson, Director, MIT FutureTech, MIT
Title: “Beyond AI Exposure: Which Tasks are Cost-Effective to Automate with Computer Vision?“
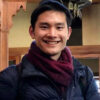
Hong-Yi TuYe, PhD Candidate, MIT Sloan
Title: “Early Experimental Evidence on the Behavioral Dynamics of Prompt Engineering”
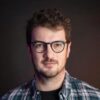
Luca Vendraminelli, Post-Doctoral Researcher, Stanford University
Title: “When Following the Domain Experts Doesn’t Capture Expertise: The Interplay Between Task and Organizational Structure in Impacting AI Development and Use”
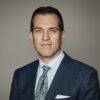
Michael Vennera, EVP & Chief Strategy, Corporate Development & Information Officer at Independence Blue Cross (IBC)
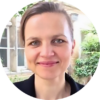
Emmanuelle Walkowiak, Vice-Chancellor’s Senior Research Fellow, RMIT University
Title: “Generative AI, O-ring Risks and Work“
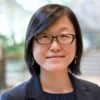
Jing Wang, Head of Center for Analytics and Insights at Vanguard
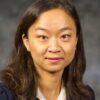
Lei Wang, Assistant Professor of Information Systems, Penn State University, Smeal College of Business
Title: “The Double-Edged Roles of Generative AI in the Creative Process: Experiments on Design Work”
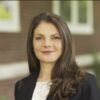
Nataliya Wright, Assistant Professor of Business, Columbia Business School
Title: “Does AI Cheapen Talk? Evidence From Global Startup and Hiring Contexts“
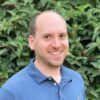
Doron Yeverechyahu, PhD student, Coller School of Management, Tel Aviv University
Title: “The Impact of Large Language Models on Open-Source Innovation: Evidence from GitHub Copilot“
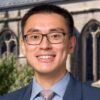
Victor Yifan Ye, Research Fellow, Stanford University
Title: “Simulating Endogenous Global Automation“
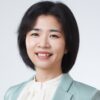
Di Yuan, Post-Doctoral Fellow, Gies College of Business, UIUC
Title: “Backfiring AI? AI Deployment in Workplace”
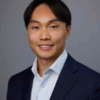
Eric Zhou, PhD student, Boston University, Questrom School of Business
Title: “Who Expands the Creative Frontier with Generative AI”
Poster Session Speakers
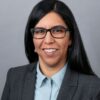
Sarah Bana, Assistant Professor of Management Science, Chapman University
Title: “The Impacts of Generative AI on Software Development Productivity”
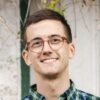
Nicholas Emery-Xu, PhD student, Department of Economics, UCLA
Title: “Economic impacts of AI-augmented R&D“
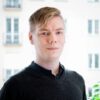
Mark Hellsten, PhD Candidate, Aarhus University
Title: “Returns to AI skills”
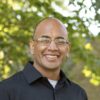
Thomas Heverin, Teacher Cybersecurity and Ethical Hacking, The Baldwin School
Title: “AI Empowered Novices: From Rational Decisions to Intuitive Decisions to Real World Impacts in Cybersecurity”
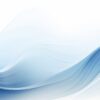
Mengyao Huang, PhD student, Berkeley Haas
Title: “Does Generative AI Lead to Better and More Equal Performances? Evidence from Crowdfunding Platforms”
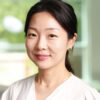
Jaeyeon Jeong, PhD student, Fox School of Business, Temple University
Title: “Can Generative AI Help Humans Learn? The Ways to Use LLMs in the Learning Process”
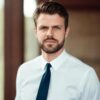
Moritz Joerling, Assistant Professor, EM Lyon Business School
Title: “Complementarity Neglect: People prefer advisors with similar abilities instead of AI with complementary abilities”
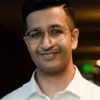
Devesh Narayanan, PhD student, Stanford University
Title: “Beyond Automation and Augmentation: Examining the Competing Moral Visions of AI in the Workplace”