News
Dozens of Researchers Present at Wharton’s Inaugural AI and the Future of Work Conference
On May 22 and 23, Wharton Human-AI Research hosted its first-ever AI and the Future of Work Conference at the Wharton School. This two-day conference saw hundreds of industry and academia members gather to hear from more than 40 leading experts on how artificial intelligence is transforming the workplace.
Nancy Rothbard, Deputy Dean and David Pottruck Professor of Management at the Wharton School, leaned into the conference’s theme by utilizing ChatGPT to help write part of her opening remarks. “Over the next few days, we’ll delve into the challenges and opportunities AI presents,” Rothbard said with a wry smile, “fostering discussions that will shape the future of work in the age of intelligent technologies.”
Knowing audience members got a chuckle out of this particular choice of words. As language models evolve and their output becomes more indistinguishable from human writing, the persistence of “delve” in responses generated by ChatGPT remains a telltale indicator of AI involvement. Wharton professor Ethan Mollick would later explain this is likely a function of AI models being sent to Nigeria for human-supervised reinforcement learning; “delve” is much more commonly used in Nigerian English.
“In all seriousness, here are my non-AI-generated remarks,” said Rothbard, whose work focuses on the workplace and the ways it transforms. “We are at a pivotal moment in the adoption of technologies. This is a speed and scale that is truly unprecedented. This exciting time promises to reshape industries and the nature of work itself.”
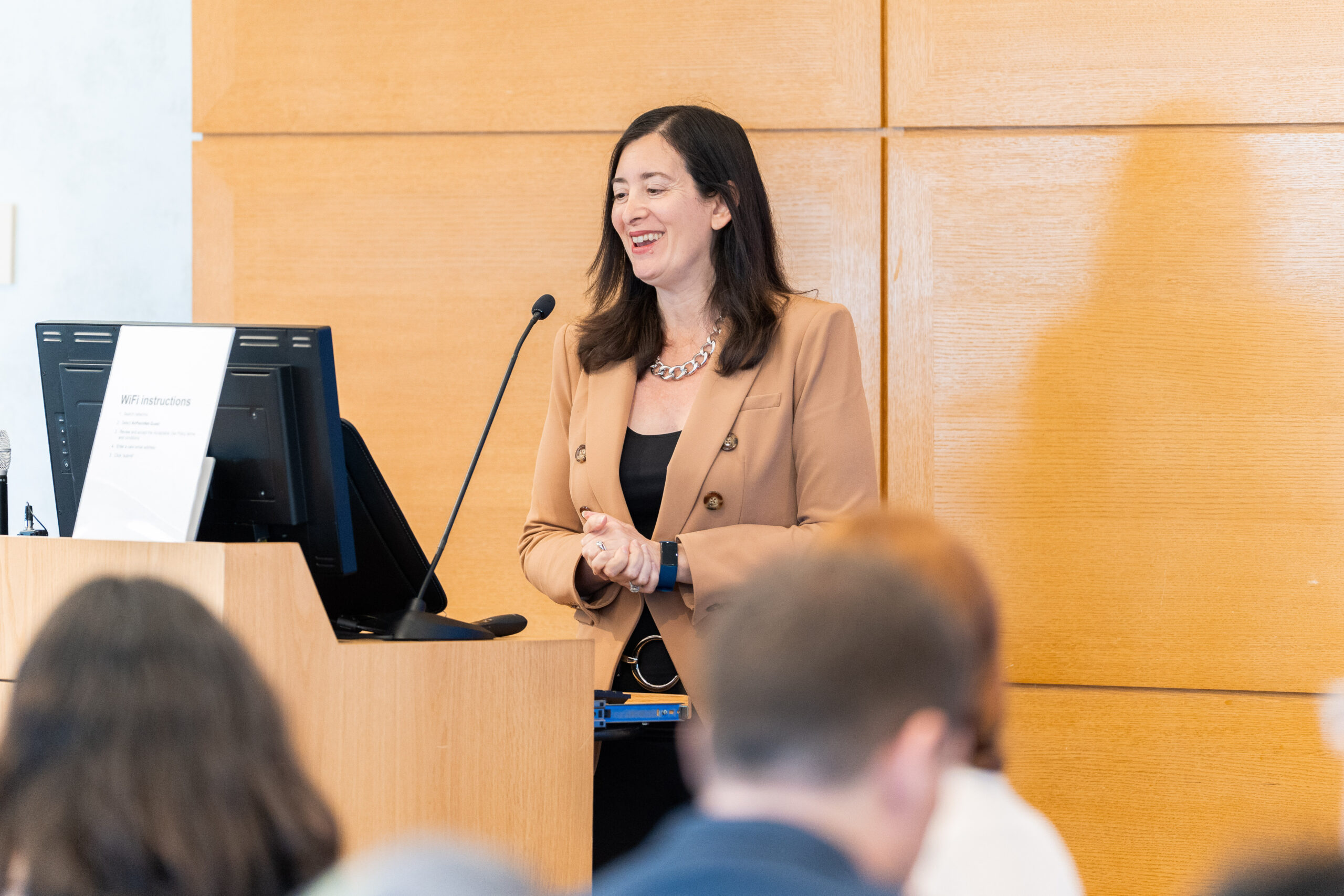
Academic Writing Will Never Be the Same
Following Rothbard, Mollick then presented the conference’s first keynote, which examined our future relationship with AI across four main topics: how we write and publish, how we research, what we research, and what we are supposed to do.
“Think about how much we use quality of writing as a screen,” he said. “We use the number of words you write as an indicator of how much you care and the quality of the words as an indicator of how smart you are. None of that matters anymore. All the writing will look good on its own face value, and we’re going to get a flood of it. Our current peer review model is not going to hold up.”
Mollick stressed academics need to establish their own standards and their own framework for the acceptable and responsible use of these tools. We, collectively, have a limited amount of time to act while these tools are still in their infancy, and it is critical that we determine the most effective and responsible means of implementation while we can.
What Comes Next for GenAI?
Tom Mitchell, Founders University Professor at Carnegie Mellon University, capped the first day of the conference with the second keynote, where he presented the four emergent trends he has noticed in the large language models (LLM) space. The first trend Mitchell identified was software plugins for LLMs, citing calculator plugins for ChatGPT as an example.
ChatGPT and similar LLMs are trained on human language and content written on the internet. Because people don’t often write complex math equations in articles or on message boards, ChatGPT had, initially, a limited understanding of certain mathematical concepts. Now, Mitchell says, ChatGPT is a reliable source for mathematical output.
“ChatGPT has no problem at all multiplying very large numbers because it doesn’t,” he said. “It calls the calculator, and it doesn’t make mistakes.”
The second trend presented by Mitchell represents an inverse of the first – rather than LLMs incorporating external plugins, existing ecosystems – like the iOS operating system on Apple devices – are now introducing LLMs into their functionality. While these developments represent exciting possibilities for the expansion of existing features, like Siri and search capabilities, they raise significant concerns regarding data and privacy.
Trends three (personal LLMs built for a given company or individual) and four (smaller, more specialized open-source models) may yield the most significant results for research and advancement in the field of AI.
Due to the massive scale and costs required to build and operate LLMs, and the tight secrecy surrounding their training data, they might not be the best route for studying AI models and researching their effects. Mitchell predicts that academics and researchers will need to find ways to examine how smaller LLM packages and AI software interact with each other in specialized roles to really make academic headway.
“There will be two orders of magnitude more researchers who are going to be looking into novel, innovative ways of combining or building societies of intelligence,” he said. “That to me is a really important trend.”
Taking AI Beyond Productivity
Mary Purk, Executive Director of Wharton Human-AI Research, hosted an industry panel with Sue Cantrell, Human Capital Eminence Leader, Vice President of Products, Workforce Strategies at Deloitte Consulting LLP, Michael Vennera, Executive Vice President & Chief Strategy, Corporate Development & Information Officer at Independence Blue Cross, and Bola Ajayi, Director of Data Science at Vanguard, about the different ways their organizations have implemented and prepared for artificial intelligence.
Vennera shared how Independence Blue Cross is piloting a new AI-powered interface for its customer service agents. Customer service is a sector that can sometimes experience high turnover, and teaching representatives the many technical nuances of insurance takes a significant amount of time. With this AI interface, the hope is that new and existing customer service agents alike can tap into a massive repository of contextual knowledge at a moment’s notice.
“If you’re a customer service agent and you’re talking to a member, and you have a question and you can’t remember or don’t know the answer, rather than [saying] ‘well, I’ll go look it up and I’ll call you back,’ can you use this interface to get an answer more quickly or more efficiently than you might otherwise be able to?” he explained.
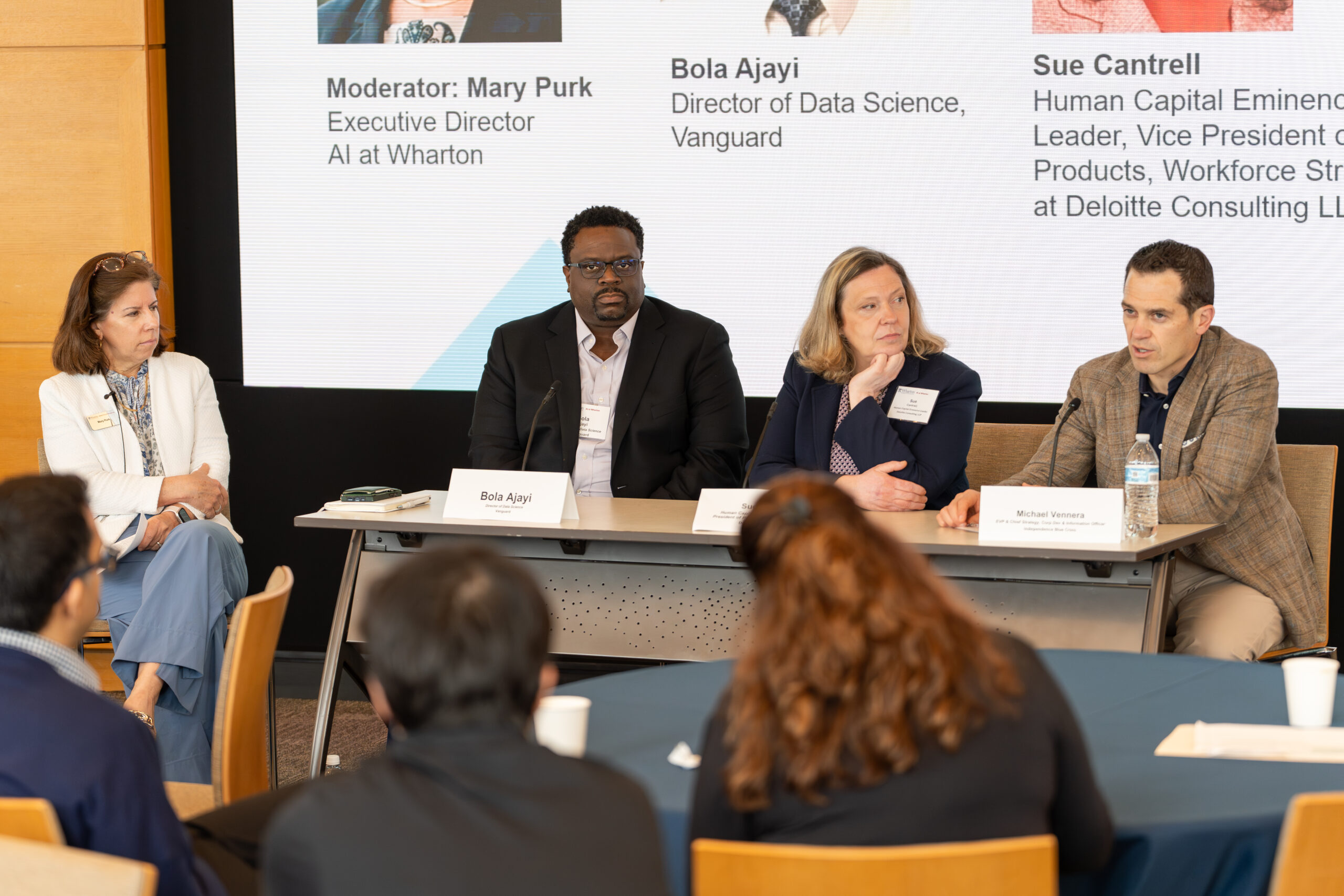
Cantrell shared an anecdote from her research about a technology company that deployed AI in a novel, worker-first way.
“They didn’t squeeze more out of working hours or monitor worker hours with AI to see who was carrying their weight and who wasn’t,” she said. “Instead, what they did was they used AI wearables combined with a mobile app to measure worker happiness, and then used AI to make suggestions on how to improve it. What they found was that productivity improved…self-confidence, and motivation improved. Ten percent improvement in profits, 34 percent improvement in sales per hour in their call centers. It’s just an example of the shift in thinking that we’ve had at Deloitte around thinking beyond productivity.”
By the end of the conference, more than 17 different sessions took place, each revealing the latest academic research into the effects of AI on the workforce. The work shared here in the infancy of modern AI will serve as an invaluable bedrock for future academic endeavors, and will help shape our relationship to this technology for years to come.
To view each session from the AI and the Future of Work Conference, click here.